Using machine learning in Mining to give the edge in exploration
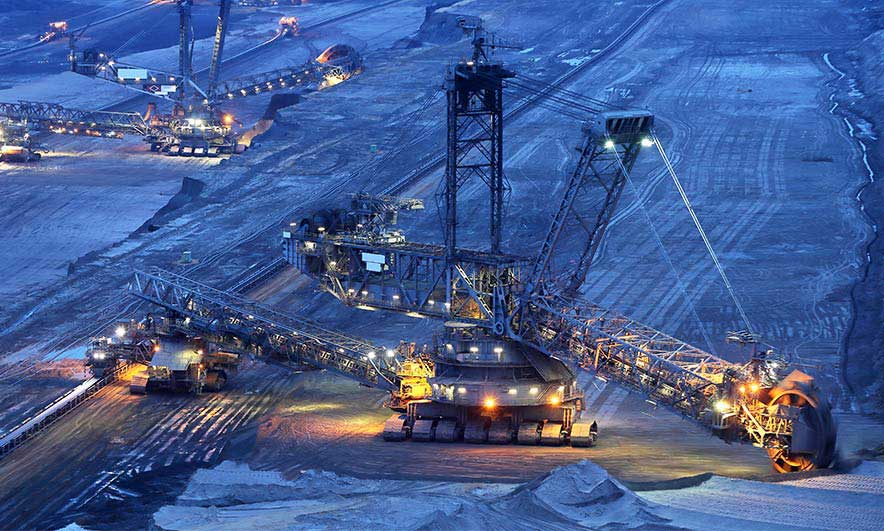
The mining industry is always seeking ways to improve the efficiency and productivity of its processes. Our appetite for new technologies often demands raw materials such as tungsten, whilst we continue to use coal-fired power stations to provide energy for our cities, despite the growing use of renewable sources. Yet these materials are increasingly rare and often challenging to extract, which means processes which are both labour-intensive and costly. Any incremental improvements can generate results that produce increased profitability, and autonomous applications, machine learning and artificial intelligence (AI) are now widely recognised as a way to achieve these results. With the additional rise of Industry and Automation 4.0, many mining companies feel encouraged to adapt and adopt the technology.
Rio Tinto, the British/Australian mining giant, were early adopters of autonomous mining systems, demonstrating exactly how well the technology improves efficiency and productivity. It began with the launch of their autonomous hauling system in 2008, moving extracted materials more efficiently and safely, creating a direct increase in productivity.
The industry has sought to improve other processes too, focusing particular interest and investment into the potential benefits of machine learning in mineral exploration. Traditional mineral exploration techniques include stream sediments geochemistry, rock and soil sampling, trenching, geophysical surveys and, of course, drilling. Mineral exploration and the accompanying exploratory drilling at identified sites carry a heavy financial burden yet the rewards for locating a rich seam are even greater. So what if machine learning could benefit this mineral exploration process
Traditional mineral exploration generates huge amounts of valuable geological data collected from equipment sensors. Companies invest hundreds of millions in geological exploration models but only utilise a small portion of the collected data. Machine learning (a form of AI) automates analytical model building using data analysis and so these systems can learn autonomously using these analytical models to identify patterns within this collected data and enable decision-making with minimal human intervention.
One company embracing the use of this BIG data is Goldspot Discoveries Inc, with an aim to “revolutionise the mineral exploration business by utilising machine learning to target on a regional and localised scale”. They pair data and geoscience experts to provide for a substantially lowered risk against an increased rate of success.
“GoldSpot Exploration’s mandate is to use robust datasets and machine learning to generate prospectivity maps.”
These maps leverage big data collected from company mining projects to display the potential likelihood of mining locations. The opportunities for prospecting are defined by the type, abundance and accessibility of the ore-grade minerals, which in turn allows better informed investment into exploration. They cite the Quebec Abitibi gold reserves as an example of their success with machine learning data analysis, having identified 86% of the existing gold deposits from a survey of only 4% of the total surface area. With the very real time and cost savings that can be made from such results, many more mining companies are now investing in an greater data scientific approach such as this.
Whilst the traditional image of mining remains open pit or underground mines, with power, conveyors, and a team 'at the coal face' using drills and tools to extract the ore, like many of our industries it's now just as reliant on the control and instrumentation systems that send signals and data back to be analysed. Mining today remains big business, seeking data as much as the raw material itself. As AI continues to advance, the precision with which the mining industry sets about its work will only get greater along with the investment that supports it.